It should go without saying that NMR is an incredibly important characterization technique with profoundly broad applicability across the entirety of chemistry. Rarely do you find something that people who work on proteins and wacky main-group synthesis both consider crucial to their work. Given powerful enough magnets and high-quality samples, rich structural information can be obtained for all manner of molecules large and small. Large molecules do pose a problem with the sheer volume of information contained within a single spectrum. Because of this, there exists a need to develop computational programs that can translate spectra into detailed structural models. Currently, existing methods predict NMR spectra based on a combination of experimentally based databases with chemical shift heuristics. These simulations, while useful, lack high predictive rigor and often have difficulty simulating the messiness of real world data. This is particularly challenging because experimental spectra can often have significant chemical shift deviations from predicted values, with those peaks discarded as outliers.
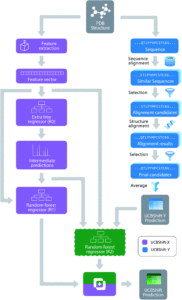
Figure 1. The overall design of the novel UCBShift chemical shift prediction algorithm, combining both a transfer prediction module a machine learning module.
To face these challenges and generate more accurate results, researchers in the US developed a new algorithm that uses both machine learning and transfer prediction (Figure 1). Transfer prediction has been widely used and relies on the similarities of NRM peak sequences between known data, typically clean datasets, and the experimental sample in question. The advantage of the new approach is that it allows for data that would previously have been dismissed as anomalous to be utilized and to give more accurate predictions. The researchers used high-quality datasets that they modified for accuracy. In particular, they retained the water and ligand molecules that co-crystallized with the proteins that would likely be associated with the solvated forms of the proteins. As the interactions of these small molecules can alter the spectral shifts of NMR peaks, their inclusion increases the likelihood that peaks previously considered outliers will be incorporated and analyzed.
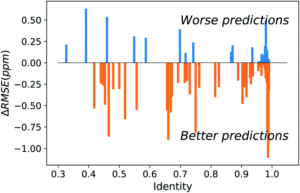
Figure 2. Difference between UCBShift-Y and SHIFTY+ (previous method) showing that overall the new algorithm is making better predictions.
Initial analysis with the new dataset produced some anomalous results, which were then mitigated by removing paramagnetic and other outlier proteins that would bias the results against the earlier algorithms. Once those were removed, the new algorithm still outperformed prior methods (Figure 2). While these advances are extremely useful for current researchers, they are approaching the limit of accuracy for systems that rely heavily on transfer predictions. In order to generate fully accurate models and structures intense work on combining deep learning with human expertise is necessary.
To find out more, please read:
Accurate prediction of chemical shifts for aqueous protein structure on “Real World” data
Jie Li, Kochise C. Bennett, Yuchen Liu, Michael V. Martin and Teresa Head-Gordon
Chem. Sci., 2020,11, 3180-3191
About the blogger:
Dr. Beth Mundy is a recent PhD in chemistry from the Cossairt lab at the University of Washington in Seattle, Washington. Her research focused on developing new and better ways to synthesize nanomaterials for energy applications. She is often spotted knitting in seminars or with her nose in a good book. You can find her on Twitter at @BethMundySci.