Quantitative prediction of material function based on multi-scale modeling is of vital importance for not only systematic performance optimization, but also precise materials design. Due to the nonintuitive and nontrivial structure-property relationship across different length scales, a comprehensive characterization of the hierarchical behavior of functional materials still remains a formidable challenge to date. To deal with this burning issue, recently Hernandez et al. made full use of data science techniques and developed an interpretable neural network architecture, viz. NestedAE, to link and quantify material properties across various length scales.
In NestedAE, an autoencoder is used to represent each physical scale of the materials, and a series of autoencoders are connected. Thus, the successive transfer of ‘‘important’’ information from one scale to another can be realized by the latent space of each autoencoder. In contrast to the previous approaches, in NestedAE each autoencoder possesses a different architecture and it is trained upon its own data set. Moreover, both the latents from the previous autoencoder and the features from the data set are reconstructed by the autoencoder.
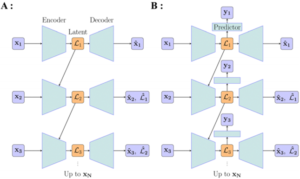
Figure 1: Unsupervised (A) and supervised (B) NestedAE architecture. Reproduced from DOI: 10.1039/d3mh01484c with permission from the Royal Society of Chemistry
To demonstrate the applicability of this newly developed machine learning architecture, Hernandez et al. employed NestedAE to compute the density-functional-theory bandgaps of metal halide perovskites based on their atomic and ionic properties. Furthermore, their power conversion efficiencies were also predicted. It was proven that the predicted results agreed well with the previous experimental observations, and its application on the metal halide perovskites established the correlation between the fundamental atomistic-level structural properties and the macroscopic device performance.
In summary, this computational study developed an interpretable machine learning architecture, NestedAE, to quantitatively predict the material properties at many length scales and to correlate the basic chemical structure and the macroscopic performance. These insightful results pioneer a new way for hierarchically optimizing and designing new functional materials.
To find out more, please read:
NestedAE: interpretable nested autoencoders for multi-scale materials characterization
Nikhil Thota, Maitreyee Sharma Priyadarshini, and Rigoberto Hernandez
Mater. Horiz., 2024, Advance article, DOI: 10.1039/D3MH01484C
About the blogger
![]()
Wen Shi is currently an Associate Professor at the School of Chemistry, Sun Yat-sen University and he is a Materials Horizons Community Board member. He received his Ph.D. in physical chemistry from Tsinghua University in 2017. From 2017 to 2021, he worked as a scientist at Institute of High Performance Computing (IHPC), Agency for Science, Technology and Research (A*STAR) in Singapore. Dr. Shi’s current research interests are in theoretical computations and simulations of functional materials. |