Neuromorphic computing, inspired by the structure of the human brain, aims to overcome the limitations of traditional computing architectures by more closely integrating processing and memory functions. It is believed that this approach is a step towards dramatically improving the efficiency of artificial neural networks by in-memory computing. Specifically, compared to conventional graphics processing units, the memristor crossbars connected by synapses would markedly enhance the training and inference of the artificial neural networks in terms of speed and power for natural language processing, image classification, and so forth.
Due to their tantalizing properties, including unique control of ionic processes, mechanical flexibility, and low cost processability, organic materials, such as small molecules, polymers, graphene oxides, and halide perovskites, have sparked considerable research interests for crossbar memristive materials for artificial neural networks. Nevertheless, some weaknesses, such as dissatisfactory environmental stability, irreproducible switching behavior, and lack of understanding of the switching mechanisms, inevitably limit their applications for such application. Thus, it is of vital importance to rationally design new organic materials for crossbar memristors and synapses, to thoroughly understand their mechanisms, and to characterize their performance.
Recently, Vishwanath et al. designed, fabricated, and evaluated one-dimensional halide perovskites for crossbar memristive materials for application in artificial neural networks. They synthesized two kinds of one-dimensional halide perovskites, one with the organic cation of propylpyridinium and the other with the organic cation of benzylpyridinium. The substituted pyridines were used as templating agents to construct the one-dimensional structures. Compared to the three-dimensional perovskites, these exhibit better resistive switching performance due to their larger band gaps.
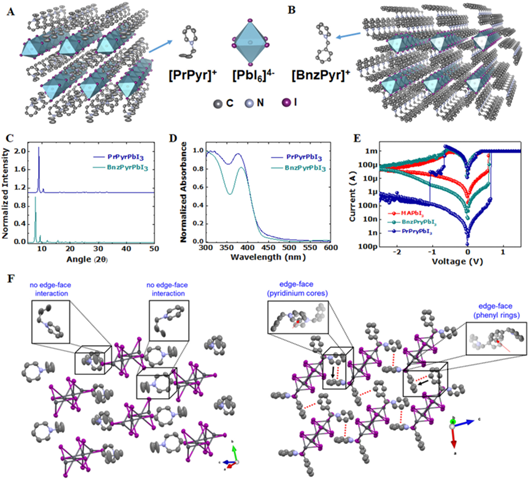
Figure 1: Comparative evaluation of 1D halide perovskites (PrPyr)[PbI3] and (BnzPyr)[PbI3]. Single crystal X-ray structures of 1D lead-iodide hybrids (A) (PrPyr)[PbI3] and (B) (BnzPyr)[PbI3]. Grey, blue, and purple spheroids represent C, N, and I atoms, respectively, while the cyan octahedron represents the [PbI6]−4 coordination sphere. Insets show the molecular structures of PrPyr+ and BnzPyr+ cations. H atoms are omitted for clarity. Thermal ellipsoids are shown at 50% probability. (C) Glancing angle X-ray diffraction (GAXRD) patterns, (D) UV-vis absorption spectra, and (E) I–V characteristics demonstrating the resistive switching effect in three different perovskites, MAPbI3, (PrPyr)[PbI3] and (BnzPyr)[PbI3]. (F) Crystal structure of (BnzPyr)[PbI3] where the edgeto-face type π-stacking interactions of aromatic cores are highlighted with dashed lines within the organic galleries. The square insets show a view down the axis from the perspective of eclipsed aromatic cores (viewing direction is denoted by the black arrows, while the red arrows point to the C atoms containing C–H ‘‘H-bond donor’’ functionalities). Reproduced from DOI: 10.1039/d3mh02055j with permission from the Royal Society of Chemistry.
Furthermore, they comprehensively analyzed the analog programming window for the halide perovskites. Concurrently, a spiking neural network with halide perovskite synapses was trained to classify the handwritten digits from the Modified National Institute of Standards and Technology database, which corroborates the applicability of the spike-timing-dependent plasticity learning of one-dimensional halide perovskite memristive synapses.
In summary, this novel study pioneers a new path for high-performance neuromorphic computing with innovative halide perovskites as the active material. These insightful results not only offer a solid foundation for the future explorations of halide perovskites in state-of-the-art neuromorphic computing, but also highlight the significance of materials innovation in unlocking the potential of next-generation computing technologies.
To find out more, please read:
High-performance one-dimensional halide perovskite crossbar memristors and synapses for neuromorphic computing
Sujaya Kumar Vishwanath, Benny Febriansyah, Si En Ng, Tisita Das, Jyotibdha Acharya, Rohit Abraham John, Divyam Sharma, Putu Andhita Dananjaya, Metikoti Jagadeeswararao, Naveen Tiwari, Mohit Ramesh Chandra Kulkarni, Wen Siang Lew, Sudip Chakraborty, Arindam Basuf and Nripan Mathews
Mater. Horiz., 2024, Advance Article, DOI: 10.1039/d3mh02055j
About the blogger
![]()
Wen Shi is currently an Associate Professor at School of Chemistry, Sun Yat-sen University and a Materials Horizons Community Board member. He received his Ph.D. in physical chemistry from Tsinghua University in 2017. From 2017 to 2021, he worked as a scientist at Institute of High Performance Computing (IHPC), Agency for Science, Technology and Research (A*STAR) in Singapore. Dr. Shi’s current research interests are in theoretical computations and simulations of functional materials. |