Developing low-cost, earth-abundant catalysts is essential in the quest for green hydrogen production through electrochemical water splitting. However, screening and optimizing the performance of these materials has traditionally been a time-consuming and resource-intensive process. Integrating innovative approaches such as machine learning in electrocatalysis presents a promising solution to expedite catalyst screening and discovery. Could these strategies be the game-changer in electrocatalysis design and testing? A recent study by Lim et al. suggests that combining machine learning with lab automation is ideal for identifying effective catalysts for hydrogen and oxygen evolution reactions.
The central figures of this study are transition metal layered double hydroxide (LDH) catalysts known for their unique lamellar structure and tunable chemical compositions. By examining five metal components (Ni, Co, Fe, Mo, and W) at varying ratios, the study efficiently leveraged machine learning to explore different compositions and correlate experimental electrochemical performance. This approach followed a simple yet powerful machine learning optimization workflow (Figure 1) that utilized a small initial dataset, Bayesian Optimization, and three machine learning algorithms: the Gaussian Process Regression, Gradient Boosting, and Neural Networks.
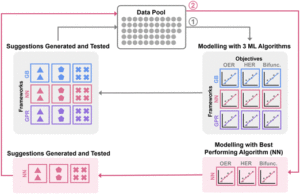
Figure 1: Summary of machine learning optimization workflow. Reproduced from DOI: 10.1039/D3MH00788J with permission from the Royal Society of Chemistry.
The neural networks proved most effective in predicting optimal catalyst compositions. The champion catalyst emerged as the molybdate-intercalated CoFe LDH, exhibiting overpotentials of 266 and 272 mV for the oxygen and hydrogen evolution reactions, respectively, while maintaining a decent stability over 50 hours. What makes this particular combination stand out? Integrating molybdate is thought to disrupt the LDH’s turbostratic structure, thereby increasing the number of active sites. Interestingly, the study also noted an unexpected outcome: Ni, typically a critical component in high-performing water-splitting electrocatalysts, was frequently excluded by the model’s recommendations. Why does this occur? It is time for the electrocatalysis detectives to investigate!
An automated synthesis system also provided an effective platform for scaling up these materials without significantly altering their physical and chemical properties. This aspect highlights the potential for industrial application and sets a precedent for scaling up electrocatalytic materials in the field.
In summary, this study underscores the potential of integrating machine learning methods into experimental workflows. This approach expedites the optimization of electrocatalysis performance, marking a substantial advancement in developing efficient and sustainable hydrogen production technologies.
To find out more, check out the full publication:
Machine learning-assisted optimization of multi-metal hydroxide electrocatalysts for overall water splitting
Carina Yi Jing Lim, Riko I Made, Zi Hui Jonathan Khoo, Chee Koon Ng, Yang Bai, Jianbiao Wang, Gaoliang Yang, Albertus D. Handoko, and Yee-Fun Lim
Mater. Horiz., 2023,10, 5022-5031 DOI: 10.1039/D3MH00788J
About the blogger
![]()
Raul A. Marquez is a Chemistry Ph.D. student working with Prof. C. Buddie Mullins at The University of Texas at Austin and a Materials Horizons Community Board member. His research focuses on understanding the chemical transformations of electrocatalytic materials and developing functional devices for energy storage and conversion technologies. Follow Raul’s latest research by following him on X (formerly Twitter): @ruloufo |