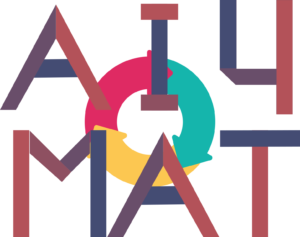
We are pleased to announce that we are partnering with the AI for Accelerated Materials Design “AI4Mat” workshop at NeurIPS 2023. Digital Discovery will be publishing selected submissions from this exciting workshop in an upcoming special article collection, to be publicised in early 2024.
The AI for Accelerated Materials Discovery (AI4Mat) Workshop 2023 provides an inclusive and collaborative platform where AI researchers and material scientists converge to tackle the cutting-edge challenges in AI-driven materials discovery and development. Its goal is to foster a vibrant exchange of ideas, breaking down barriers between disciplines and encouraging insightful discussions among experts from diverse disciplines and curious newcomers to the field. The workshop embraces a broad definition of materials design encompassing matter in various forms, such as crystalline and amorphous solid-state materials, glasses, molecules, nanomaterials, and devices. By taking a comprehensive look at automated materials discovery spanning AI-guided design, synthesis and automated material characterization, the organisers aim to create an opportunity for deep, thoughtful discussion among researchers working on these interdisciplinary topics, and highlight ongoing challenges in the field.
Find out more about the workshop, including submission guidelines, at the web site. Details of the Digital Discovery article collection will be shared with the participants in due course.